Unlocking the Power of Medical Datasets for Machine Learning
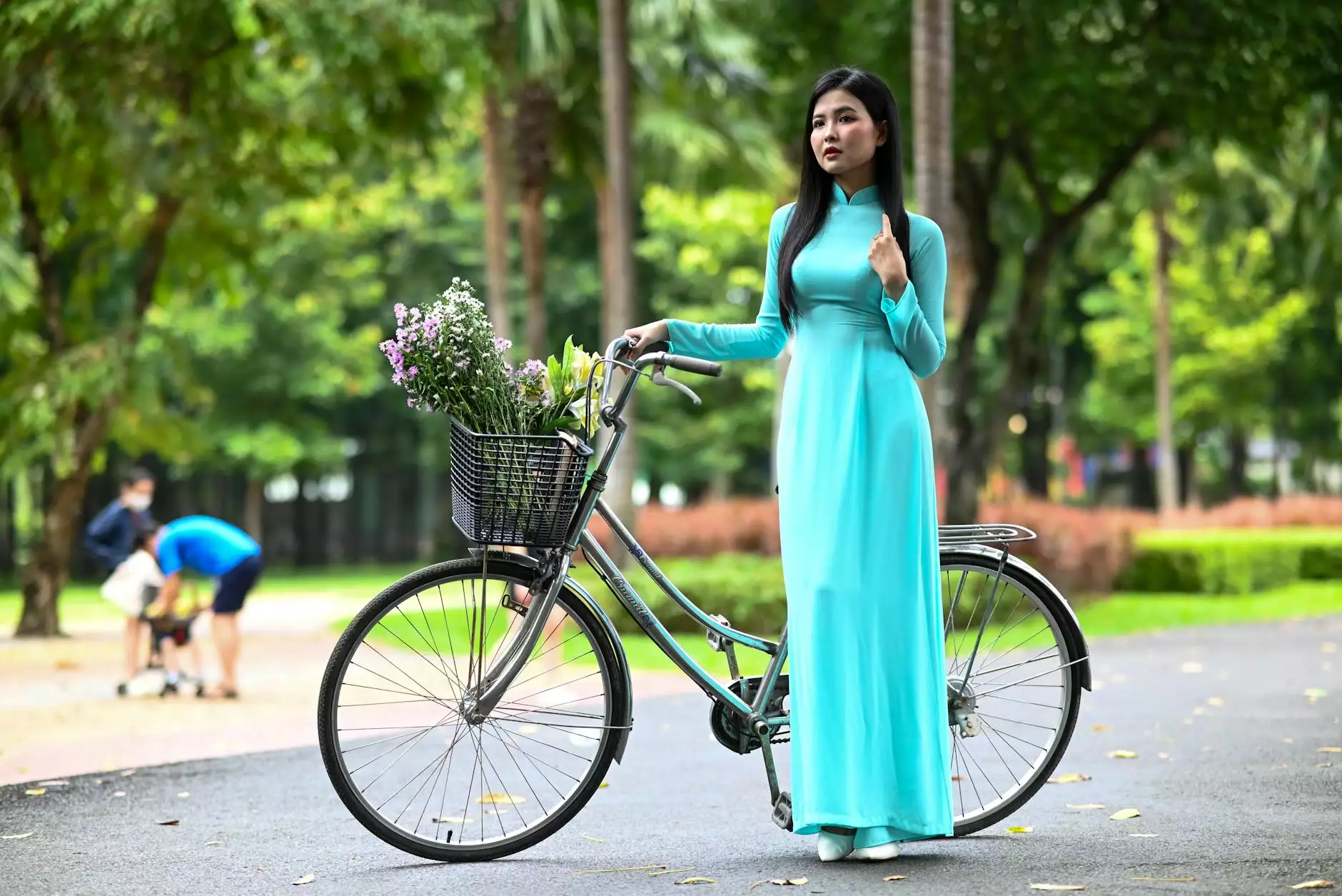
In today’s rapidly advancing technological landscape, the integration of machine learning into the medical field is transforming how healthcare providers deliver services and improve patient outcomes. Central to this transformation is the utilization of medical datasets for machine learning, which play an essential role in developing predictive models, informing clinical decisions, and ultimately, enhancing patient care.
Understanding Medical Datasets
Medical datasets consist of structured and unstructured data collected from various healthcare sources, including patient records, clinical trials, imaging studies, and health surveys. These datasets can contain demographic data, medical histories, treatment plans, and outcomes, which are vital for training machine learning models.
The Importance of Quality Data
The efficacy of machine learning in healthcare hinges on the quality of the datasets used. High-quality datasets are marked by:
- Completeness: Datasets must include comprehensive information to avoid biases in machine learning algorithms.
- Accuracy: Erroneous data can lead to incorrect model predictions, which can have dire consequences in a medical setting.
- Consistency: Data should be uniform across sources to ensure reliable analysis.
- Timeliness: Recent data tends to provide better insights, reflecting current trends in patient health and treatment efficacy.
Applications of Machine Learning in Healthcare
The application of machine learning powered by medical datasets is vast. Here, we delve into some of the most impactful uses:
1. Predictive Analytics
Machine learning algorithms analyze historical healthcare data to predict future outcomes. For instance, hospitals leverage these models to forecast patient admission rates, optimize staffing, and manage resources effectively. Predictive analytics can significantly enhance operational efficiency.
2. Personalized Medicine
Using data from genetic studies and patient records, machine learning can tailor treatments to individual patients' needs. This personalized medicine approach increases the likelihood of successful outcomes and minimizes unnecessary side effects.
3. Improved Diagnostics
Machine learning systems can analyze medical images and biological data to identify diseases at earlier stages than traditional methods. For instance, algorithms trained on imaging datasets can detect tumors with remarkable accuracy, aiding radiologists in their assessments.
4. Drug Development
Pharmaceutical companies harness machine learning algorithms to analyze chemical compounds and their effects. By leveraging medical datasets, they can uncover relationships and streamline the process of drug discovery, ultimately bringing life-saving medications to market faster.
Challenges in Utilizing Medical Datasets
Despite the promise of machine learning and its applications in healthcare, several challenges persist, including:
1. Data Privacy Concerns
Healthcare data is sensitive, and strict regulations like HIPAA in the United States protect patient information. Collecting, sharing, and analyzing data while maintaining confidentiality remains a significant hurdle.
2. Bias in Data
If medical datasets do not accurately represent all populations, machine learning models may perpetuate existing biases and health disparities. It’s crucial to ensure that datasets are diverse and comprehensive.
3. Integration with Existing Systems
Integrating machine learning solutions into existing healthcare systems can be technically challenging and often requires significant investment in technology and training.
Case Studies: Success Stories in Healthcare
Several healthcare providers and technology companies have successfully implemented machine learning using medical datasets, leading to improved patient outcomes. Here are some notable case studies:
1. IBM Watson Health
IBM Watson Health employs machine learning algorithms to analyze vast quantities of medical data. Its capabilities in oncology help oncologists identify treatment options tailored to each patient's unique genetic profile, enhancing the effectiveness of cancer treatment.
2. Google Health's Diabetic Retinopathy Screening
Google Health developed machine learning algorithms capable of analyzing retinal images to detect diabetic retinopathy. Using extensive medical datasets, the system achieved accuracies comparable to human experts, offering an essential tool for early diagnosis.
The Future of Medical Datasets in Machine Learning
The future of medical datasets for machine learning looks promising. As technology evolves, several trends are expected to shape its trajectory:
1. Enhanced Data Sharing
Efforts to standardize data formats and promote secure data sharing will pave the way for broader access to medical datasets, facilitating collaborative research and innovation.
2. Real-Time Data Analytics
With the rise of wearable technology and IoT devices, real-time data collection will allow healthcare providers to monitor patients proactively and intervene earlier, improving overall health management.
3. Focus on Patient-Centric Models
The shift towards patient-centric care will drive the development of machine learning models that are more attuned to the individual patient experience, ultimately empowering patients in their healthcare journey.
Conclusion: Embracing the Future of Healthcare
In conclusion, the integration of medical datasets for machine learning represents a revolutionary shift in healthcare practices. By leveraging these datasets, healthcare providers can enhance diagnostics, personalize treatment plans, and streamline operations. As the medical community continues to embrace technological advancements, the prospects for improved patient care and greater efficiency in healthcare systems are boundless.
For businesses in the healthcare sector, investing in machine learning capabilities and focusing on building robust medical datasets will not only drive innovation but also foster a culture of continuous improvement. The future of healthcare is here, and it is powered by data.
Key Takeaways
- Quality medical datasets are essential for effective machine learning applications.
- The potential applications include predictive analytics, personalized medicine, improved diagnostics, and drug development.
- Addressing challenges such as data privacy, bias, and integration is critical for success.
- Success stories demonstrate the transformative impact of these technologies on patient care.
- The future will see enhanced data sharing, real-time analytics, and patient-centric models emerging as key trends.