Revolutionizing Healthcare: The Importance of Medical Data Annotation
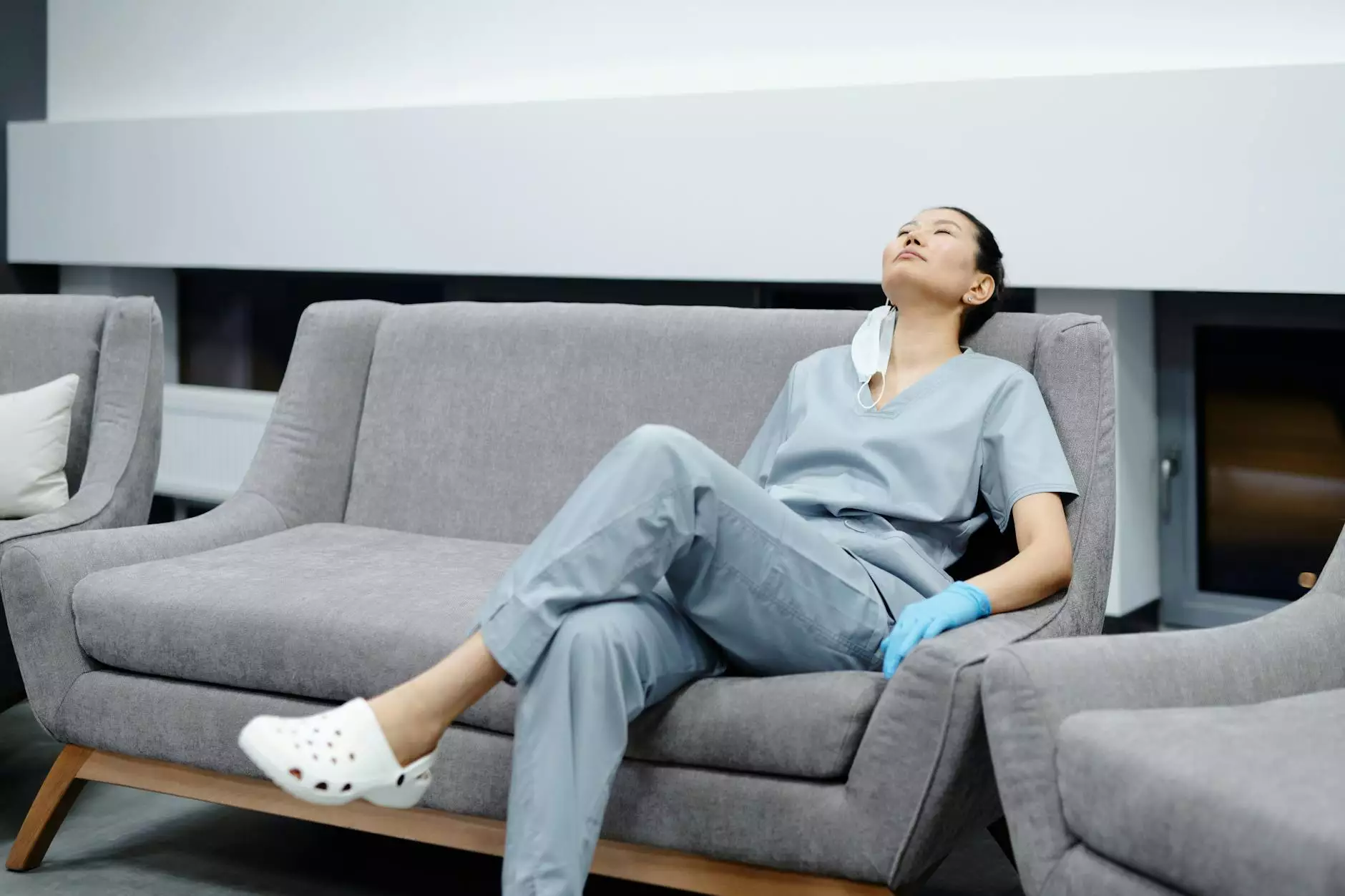
In recent years, the healthcare industry has seen a significant transformation fueled by advancements in artificial intelligence (AI) and machine learning (ML). A critical component of this transformation is medical data annotation, a process that involves the meticulous labeling and categorization of medical data to enhance the accuracy and efficiency of AI applications in healthcare. This article delves into the significance of medical data annotation, exploring its role in the healthcare ecosystem, the techniques involved, the challenges faced, and the future trends that could shape its landscape.
What is Medical Data Annotation?
Medical data annotation refers to the process of labeling medical data—such as images, texts, and videos—so that AI algorithms can learn from these labeled datasets. This practice is crucial for training machine learning models to recognize patterns, identify anomalies, and ultimately provide better diagnostic insights and treatment options. The accuracy of AI predictions largely depends on the quality of annotated data, making this process a linchpin in the development of reliable healthcare solutions.
The Types of Medical Data Commonly Annotated
There are several types of medical data that require annotation. Here are some of the most common:
- Medical Imaging: This includes X-rays, MRIs, CT scans, and ultrasound images that are annotated to help identify diseases, conditions, or abnormalities.
- Clinical Text: Electronic health records (EHRs), physician notes, and discharge summaries require annotation to extract relevant information and improve patient care.
- Genomic Data: Annotating genomic sequences helps in identifying genetic disorders and enables personalized medicine approaches.
- Pathology Slides: Annotating histopathological slides is crucial for training algorithms that assist in cancer diagnosis.
The Significance of Medical Data Annotation in Healthcare
The implications of medical data annotation in the healthcare sector are profound. Here are some ways this process enhances healthcare delivery:
- Improved Diagnostic Accuracy: Medical data annotation allows AI systems to learn from a wide array of data inputs. By training models on well-annotated datasets, healthcare providers can achieve higher diagnostic accuracy, which is crucial in early disease detection and effective treatment protocols.
- Streamlined Workflow: By automating the analysis of medical data, annotation significantly reduces the workload on healthcare professionals, allowing them to focus on patient care rather than data processing.
- Enhanced Research Capabilities: Annotated datasets can significantly aid research efforts. Researchers can use these datasets to explore new therapeutic avenues, understand disease progression, and conduct clinical trials with greater ease.
- Personalized Patient Care: By leveraging annotated data, practitioners can make more informed decisions, tailoring treatments based on individual patient needs and genetic profiles.
Techniques of Medical Data Annotation
The process of medical data annotation employs various techniques, each suited to different types of data and specific use cases. Below are some commonly utilized methods:
1. Image Annotation
This involves outlining or marking regions of interest in medical images. Techniques used can vary from simple bounding boxes to more complex semantic segmentation, which classifies each pixel in an image.
2. Text Annotation
In clinical text, annotation can include named entity recognition to identify patient names, medication, diagnoses, etc. This can help in extracting structured information from unstructured data.
3. Video Annotation
For interpreting procedural videos or patient movements, video annotation involves tagging specific actions or events, facilitating the development of AI models that can understand and improve clinical practices.
Challenges in Medical Data Annotation
Despite its advantages, medical data annotation is laden with challenges. Addressing these challenges is crucial for maximizing the benefits derived from annotated data.
- Resource Intensive: Annotating medical data can be very time-consuming and labor-intensive, requiring specialized knowledge and expertise, which can strain resources.
- Quality Control: Ensuring the accuracy and consistency of annotations is vital. Inaccurate data can lead to misleading results, making quality assurance a critical concern.
- Privacy Concerns: Handling sensitive patient data comes with ethical responsibilities. Ensuring compliance with regulations like HIPAA is paramount to avoid legal repercussions.
- Scalability: As the volume of medical data continues to grow exponentially, scaling the annotation process poses a significant challenge for healthcare institutions.
The Future of Medical Data Annotation
The future of medical data annotation appears promising, with several trends shaping its evolution:
1. AI-Driven Annotation Tools
The integration of AI in the annotation process itself is on the rise. Machine learning algorithms are increasingly being used to assist in the annotation, reducing the time and effort required while improving accuracy.
2. Crowdsourcing and Collaboration
Many organizations are turning to crowdsourcing as a solution to the resource-intensive nature of medical data annotation. By tapping into a global workforce, they can diversify their annotation efforts and complete projects faster.
3. Enhanced Training and Standards
With the growing demand for medical data annotation, there will be a considerable emphasis on developing standardized training modules that can ensure consistency and quality across different annotators and organizations.
4. Legal and Ethical Frameworks
As the reliance on data grows, the establishment of clearer legal and ethical frameworks surrounding data annotation will be critical. This includes developing guidelines for consent, data usage, and privacy protection.
Conclusion
In conclusion, medical data annotation stands at the forefront of healthcare innovation, playing a crucial role in enhancing diagnostic accuracy and patient care. As we move toward a more data-driven future in healthcare, the importance of high-quality annotated data cannot be overstated. Organizations like Keymakr are leading the way in software development solutions that focus on advancing data annotation technologies. By embracing this transformative process, we can harness the power of AI and machine learning to revolutionize healthcare and improve outcomes for patients worldwide.